AI, ML, & Ubuntu: Everything you need to know
Canonical
on 26 July 2018
Tags: AI , AI/ML , GPGPU , Kubeflow , kubernetes , ML , MLOps , TensorFlow , Ubuntu
AI and ML adoption in the enterprise is exploding from Silicon Valley to Wall Street. Ubuntu is the premier platform for these ambitions — from developer workstations, to racks, to clouds and to the edge with smart connected IoT. One of the joys that come with new developer trends are a plethora of new technologies and terminologies to understand.
In this webinar, join Canonical’s Kubernetes Product Manager Carmine Rimi for:
- An introduction to some of the key concepts in Machine Learning
- A look into some examples of how AI applications and their development are reshaping company’s IT
- A deep dive into how enterprises are applying devops practices to their ML infrastructure and workflows
- An introduction to Canonical AI / ML portfolio from Ubuntu to the Canonical Distribution of Kubernetes and and how to get started quickly with your project
And in addition, we’ll be answering some of these questions:
- What do Kubeflow, Tensorflow, Jupyter, and GPGPUs do?
- What’s the difference between AI, ML and DL?
- What is an AI model? How do you train it? How do you develop / improve it? How do you execute it?
And finally, we’ll be taking the time to answer your questions in a Q&A session
Enterprise AI, simplified
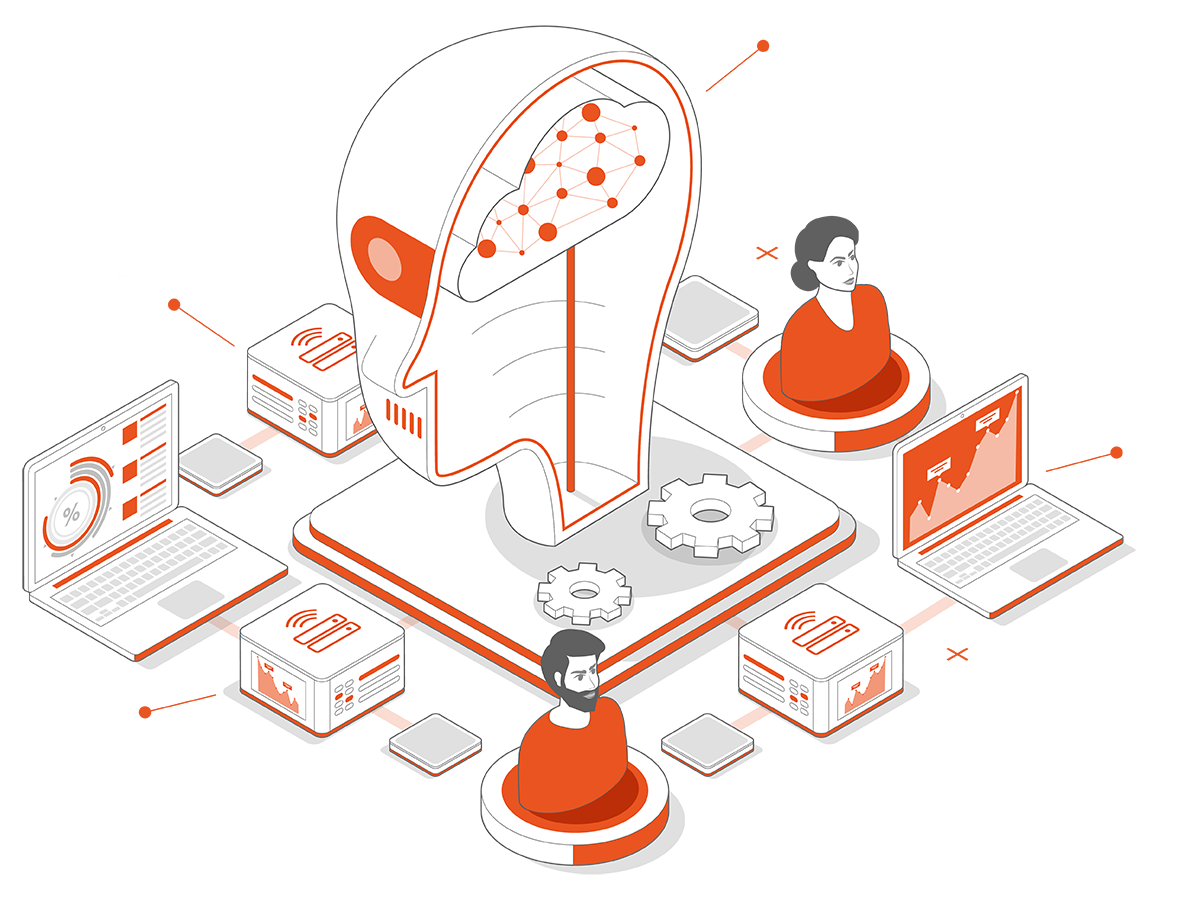
AI doesn’t have to be difficult. Accelerate innovation with an end-to-end stack that delivers all the open source tooling you need for the entire AI/ML lifecycle.
Newsletter signup
Related posts
How to deploy Kubeflow on Azure
Kubeflow is a cloud-native, open source machine learning operations (MLOps) platform designed for developing and deploying ML models on Kubernetes. Kubeflow...
Meet Canonical at KubeCon + CloudNativeCon North America 2024
We are ready to connect with the pioneers of open-source innovation! Canonical, the force behind Ubuntu, is returning as a gold sponsor at KubeCon +...
KubeCon Europe 2025: Containers & Connections with Ubuntu
It’s hard to believe that the first KubeCon took place nearly 10 years ago. Back then, Kubernetes was still in its early days, and the world was only just...